Dr James Cugley, Lecturer in Applied Engineering in the School of Engineering, Technology and Design, provides insight into the use of imaging and AI to diagnose and monitor coronavirus.
The Coronavirus Disease (COVID-19) outbreak has had a tremendous impact on global health and the daily life of people all over the world[1]. Fast and accurate testing of suspected cases is a priority in order to slow or maintain the spread of the virus. Currently the majority of tests in operation rely on physically detecting the coronavirus material within the patients nose, throat, blood sample or specimens from the lower respiratory tract[2]. These operations are all time consuming, highly human labour dependant and have been shown to have a poor detection rate. Therefore, it has become increasingly more relevent to gain a powerful method of screening and monitoring patients.
For these reasons radiological imaging is preferred where chest X-rays and CT (Computed tomography) scans have both emerged as successful tools for diagnosis[3]. Early studies express that patients with a presence of COVID-19 exipit abnormality in chest X-rays and CT images.
Previously[4], it has been presented that a combination of Imagining analysis and AI (Artifical Inteligence) such as Neural Networks known in the literature as Deep Learning could be used in order to predict whether or not a patient has a desiese and even to monitor the severity of the infection. This practice has now been used by serval researchers to diagnose and monitoring coronavirus within patients[5]–[9].
Below Figure 1 details a generic summary of the analysis techniques that could be incorporated in order to process both 2D and 3D radiological images of the patient and use this analysis to generate a ‘score’ for the amount of abnormalities present within the respiratory system that have been previously linked to coronavirus. Typically, within image analysis, areas of interest are first isolated in order to cut down on computational time, and then specific patterns are recognised by the system[10]. The area or shape of these collected details then form a data set of numerical values.
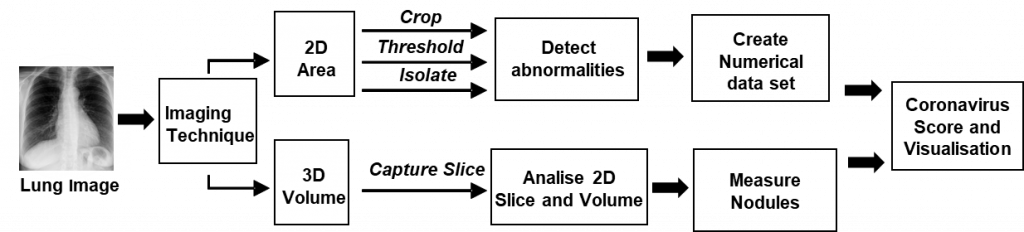
ANN (Artifical Neural Networks) models are computing systems inspired by biological neural networks[11] (a diagram of a typical neural network is shown in Figure 2.) They are built as a framework for many different machine learning algorithms. Although there are many types of artificial neural networks, the basic concept often remains the same or similar. The data set (in this instance numerical representations of the analysed images) enters through the input layer as probabilistic signals, and converts them within the hidden layer corresponding to the biological neuron’s axon and the weightings of the input. The adjusting of these weightings ‘trains’ the algorithm to learn the importance of the input parameters when trying to achieve the goal at hand, in this instance an act of classification or scoring the probability of a patient being infected with coronavirus.
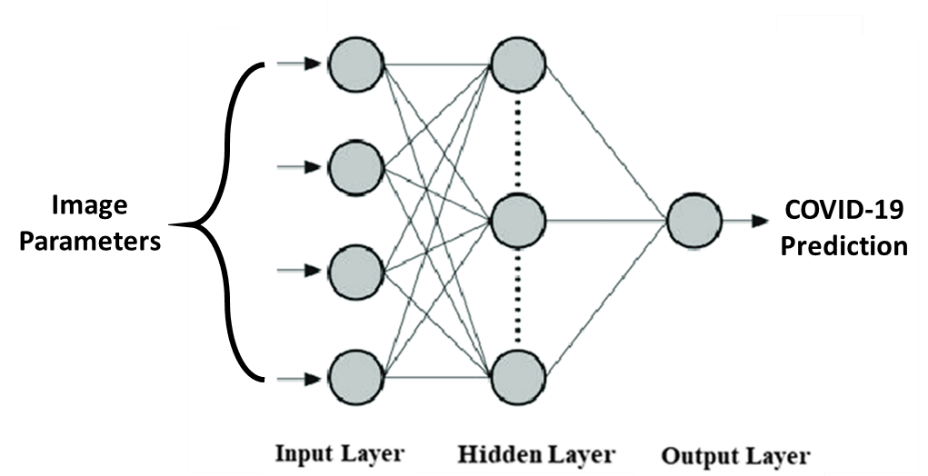
Already a number of studies have shown a high rate of accuracy when detecting COVID-19 positive patients Incorporating these systems. The authors are very confident that works like this could be the answer to an effective and safe method for evaluating the high volumes of patient screening and diagnostics during this time.
References
[1] WHO, “Coronavirus disease (COVID-19) Situation Report – 129,” World Heal. Organ., no. May, 2020.
[2] F. Yu, L. Du, D. M. Ojcius, C. Pan, and S. Jiang, “Measures for diagnosing and treating infections by a novel coronavirus responsible for a pneumonia outbreak originating in Wuhan, China,” Microbes Infect., vol. 22, no. 2, pp. 74–79, 2020.
[3] D. Zhao et al., “Early X-ray and CT appearances of severe acute respiratory syndrome: an analysis of 28 cases,” Chin. Med. J. (Engl)., vol. 116, no. 6, p. 823—826, Jun. 2003.
[4] D. S. Kermany, M. Goldbaum, W. Cai, and M. A. Lewis, “Identifying Medical Diagnoses and Treatable Diseases by Image-Based Deep Learning,” Cell, vol. 172, no. 5, pp. 1122-1131.e9, 2018.
[5] F. Ucar and D. Korkmaz, “COVIDiagnosis-Net : Deep Bayes-SqueezeNet based diagnosis of the coronavirus disease 2019 ( COVID-19 ) from X-ray images,” Med. Hypotheses, vol. 140, no. April, p. 109761, 2020.
[6] O. Gozes, M. Frid, H. Greenspan, and D. Patrick, “Rapid AI Development Cycle for the Coronavirus ( COVID-19 ) Pandemic : Initial Results for Automated Detection & Patient Monitoring using Deep Learning CT Image Analysis Article Type : Authors : Summary Statement : Key Results : List of abbreviations :,” 2020.
[7] S. Wang, B. Kang, J. Ma, X. Zeng, and M. Xiao, “A deep learning algorithm using CT images to screen for Corona Virus Disease ( COVID-19 ),” pp. 1–19, 2020.
[8] M. Farooq and A. Hafeez, “COVID-ResNet : A Deep Learning Framework for Screening of COVID19 from Radiographs.”
[9] L. Wang, Z. Q. Lin, and A. Wong, “COVID-Net : A Tailored Deep Convolutional Neural Network Design for Detection of COVID-19 Cases from Chest X-Ray Images,” pp. 1–12.
[10] V. Tyagi, “Understanding Digital Image Processing,” no. November, 2018.
[11] J. Zupan, “Introduction to Artificial Neural Network ( ANN ) Methods : What They Are and How to Use Them Introduction to Artificial Neural Network ( ANN ) Methods : What They Are and How to Use Them *.,” no. January 1994, 2014.